Estimation of intrinsic images remains a challenging task due to weaknesses of ground-truth datasets, which either are too small or present non-realistic issues.
Surreal Intrinsic Dataset (SID1)
SID is a large dataset with realistic light conditions and which can be easily adapted to different tasks addressed to the estimation of light components. It is based on Shapenet objects without textures, although they could be added. We pursue a dataset with the following properties:
-
-
- Existence of cast shadows and global light consistency
- Keeping the influence of a varying surround
- Taking advantage of using the full image information for training.
-
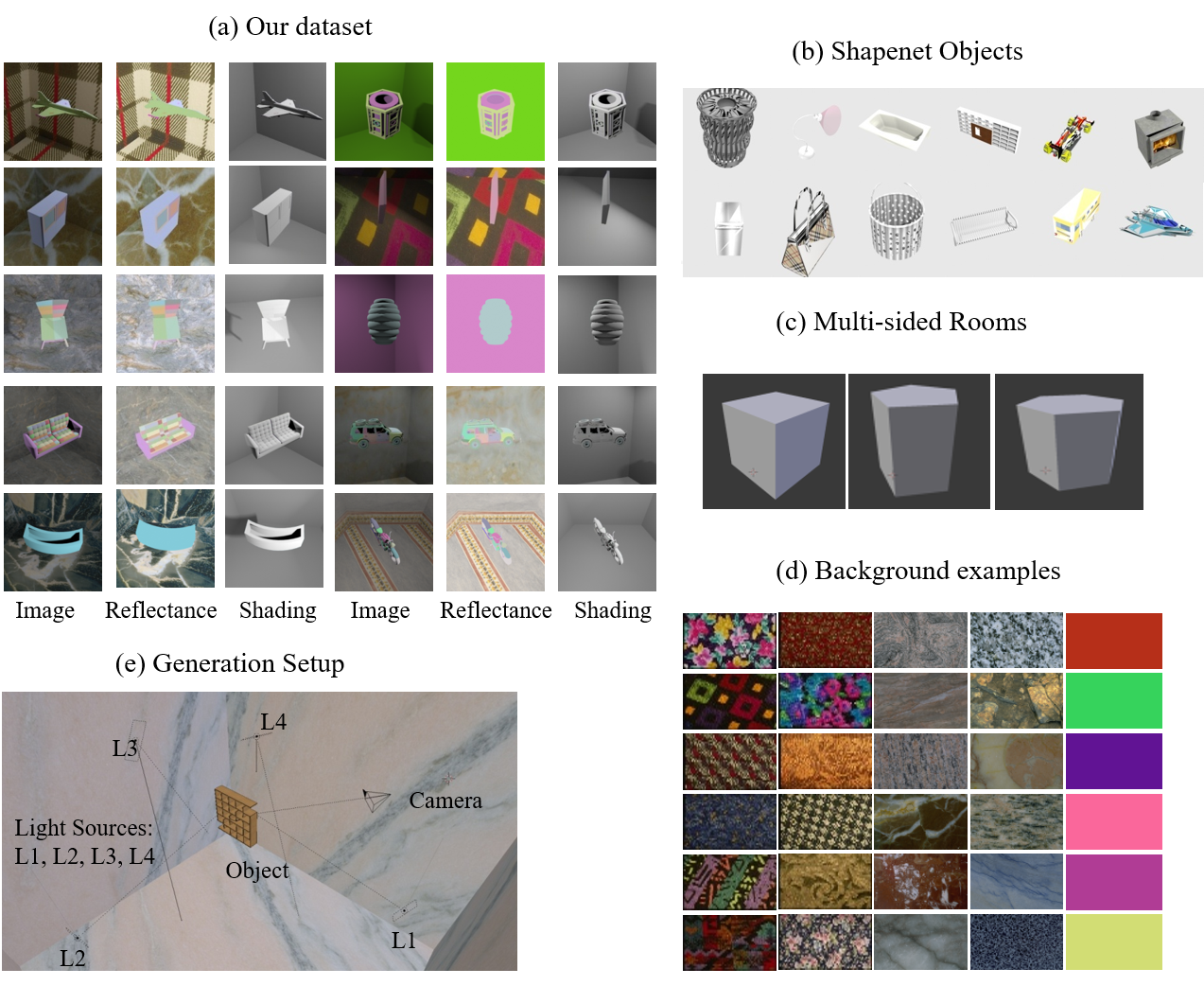
We substitute environmental maps used in Shapenet by multi-sided rooms with highly variable reflectances on the walls. The main property of environmental maps is to get diverse lighting conditions, since they allow to assume a different light source at every point of the background, but the drawback of these maps is that they are unable to cast shadows and introduce some physical inconsistencies in the light interactions of the scene. Our dataset has 25,000 ground-truth images with intrinsic data for the whole image pixels. We used the open source Blender rendering engine to generate images.
The final scenes we create are somehow surreal, since images are presenting “airplanes flying in closed rooms decorated with old-fashioned wall-papers”, but providing a wide range of realistic light effects with multiple color and shade interactions, thus we call it SID for Surreal Intrinsic Dataset.
Downloads
Send an email to hasial@cvc.uab.es to download the dataset. If you download and use the dataset for research, kindly cite the following paper.
@article{Sial:20,
author = {Hassan A. Sial and Ramon Baldrich and Maria Vanrell},
journal = {J. Opt. Soc. Am. A},
keywords = {Bidirectional scattering distribution function; Color constancy; Image metrics; Light sources; Neural networks; White light},
number = {1},
pages = {1--15},
publisher = {OSA},
title = {Deep intrinsic decomposition trained on surreal scenes yet with realistic light effects},
volume = {37},
month = {Jan},
year = {2020},
url = {http://josaa.osa.org/abstract.cfm?URI=josaa-37-1-1},
doi = {10.1364/JOSAA.37.000001},
}
Poster
Poster related to this work was presented in the Fourteenth CVC Workshop on Computer Vision Trends and Challenges (CVCRD2019)
Related Publications
-
-
- Hassan A. Sial, Ramon Baldrich, and Maria Vanrell, “Deep intrinsic decomposition trained on surreal scenes yet with realistic light effects,” J. Opt. Soc. Am. A 37, 1-15 (2020)
- Hassan A. Sial, Ramon Baldrich, Maria Vanrell, and Dimitris Samaras. “Light Direction and Color Estimation from Single Image with Deep Regression.” In London Imaging Meeting, vol. 2020, no. 1, pp. 139-143. Society for Imaging Science and Technology, 2020.
-
References